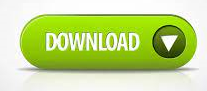

Two analytical methods are used for feature extraction, which are the static and dynamic analyses. The efficiency of these approaches differs based on the extracted features from applications, besides the algorithms used for the detection task. Machine learning methods have proved their efficiency in detecting Android malware such as the work in. More research has been focusing on developing new approaches for the detection of Android ransomware applications. Moreover, the results shows that the proposed model outperforms the state-of-the-art approaches considered in this work. The experiments show that the proposed model is efficient in detecting Android ransomware regardless of its age by achieving an accuracy of approximately 97.48%. Furthermore, the paper proposes a supervised machine learning model that is trained and tested using the diverse dataset. Moreover, this paper collected a large and diverse dataset of ransomware applications that comprises new and old ransomware developed during the period 2008–2020. This proves that supervised machine learning models trained using new ransomware dataset are inefficient in detecting old types of ransomware and vice versa. This paper sheds light on the importance of considering the age of ransomware datasets and its effects on the detection accuracy of supervised machine learning models.

Therefore, the detection accuracy for those models is inaccurate since they learned using features from specific ransomware, old or new ransomware, and they did not learn using diverse ransomware features from different ages. However, the age of the ransomware datasets was not considered when training and testing these models. These models were trained using different datasets from different sources. Several supervised machine learning models have been proposed and used to detect Android ransomware.
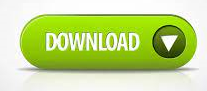